Roberta RC Saint Amour is a large language model, trained by Google. It is designed to understand and generate human-like text, and it is used in a variety of applications, including search engines, chatbots, and language translation.
Roberta RC Saint Amour is a powerful tool that can be used to improve the quality of written content. It can help to identify and correct errors, improve clarity and concision, and generate new ideas. Roberta RC Saint Amour can also be used to create new content, such as articles, blog posts, and marketing copy.
Roberta RC Saint Amour is still under development, but it has already shown great promise. It is a valuable tool for anyone who wants to improve their writing skills or create new content.
Roberta RC Saint Amour
Roberta RC Saint Amour is a large language model, trained by Google, and its key aspects include:
- Natural language processing
- Text generation
- Machine translation
- Question answering
- Summarization
- Named entity recognition
- Part-of-speech tagging
- Sentiment analysis
- Text classification
These aspects make Roberta RC Saint Amour a powerful tool for a variety of applications, including:
- Search engines
- Chatbots
- Language translation
- Content creation
- Data analysis
Natural language processing
Natural language processing (NLP) is a subfield of linguistics, computer science, and artificial intelligence concerned with the interactions between computers and human (natural) languages. As a component of Roberta RC Saint Amour, NLP is the ability of a computer program to understand and generate human language. This is a complex task, as human language is full of ambiguity and nuance. However, NLP is essential for many applications, such as search engines, chatbots, and language translation.
Roberta RC Saint Amour uses NLP to understand the meaning of text and to generate text that is both informative and engaging. For example, Roberta RC Saint Amour can be used to:
- Answer questions about the world
- Translate text from one language to another
- Summarize long documents
- Generate marketing copy
- Identify the sentiment of a piece of text
NLP is a rapidly growing field, and Roberta RC Saint Amour is at the forefront of this growth. As NLP continues to improve, Roberta RC Saint Amour will become even more powerful and versatile.
Text generation
Text generation is the ability of a computer program to create new text. This is a challenging task, as it requires the program to understand the meaning of text and to be able to generate text that is both informative and engaging. However, text generation is a key component of many applications, such as search engines, chatbots, and language translation.
Roberta RC Saint Amour is a large language model that is particularly well-suited for text generation. This is because Roberta RC Saint Amour is trained on a massive dataset of text, which gives it a deep understanding of the structure and meaning of language. As a result, Roberta RC Saint Amour is able to generate text that is both fluent and informative.
One of the most important applications of text generation is in the creation of chatbots. Chatbots are computer programs that can simulate human conversation. They are used in a variety of applications, such as customer service, technical support, and marketing. In order to be effective, chatbots need to be able to generate text that is both natural and informative. Roberta RC Saint Amour is well-suited for this task, as it can generate text that is both fluent and informative.
Machine translation
Machine translation (MT) is the use of computer software to translate text or speech from one language to another. It is a complex task that requires the computer to understand the meaning of the source text and to generate a fluent and accurate translation in the target language. However, MT is a key component of many applications, such as search engines, chatbots, and language learning tools.
- Neural machine translation
Neural machine translation (NMT) is a type of MT that uses neural networks to translate text. NMT systems are trained on large datasets of parallel text, which allows them to learn the complex relationships between languages. NMT systems are typically more accurate and fluent than traditional MT systems.
- Statistical machine translation
Statistical machine translation (SMT) is a type of MT that uses statistical models to translate text. SMT systems are trained on large datasets of parallel text, and they use statistical methods to identify the most likely translation for each word or phrase in the source text.
- Rule-based machine translation
Rule-based machine translation (RBMT) is a type of MT that uses a set of rules to translate text. RBMT systems are typically hand-crafted by linguists, and they use a variety of linguistic rules to translate text.
- Hybrid machine translation
Hybrid machine translation (HMT) is a type of MT that combines two or more different MT techniques. HMT systems are typically designed to improve the accuracy and fluency of MT output.
Roberta RC Saint Amour is a large language model that is particularly well-suited for machine translation. This is because Roberta RC Saint Amour is trained on a massive dataset of parallel text, which gives it a deep understanding of the structure and meaning of different languages. As a result, Roberta RC Saint Amour is able to generate translations that are both accurate and fluent.
Question answering
Question answering (QA) is a subfield of natural language processing (NLP) that deals with the task of automatically answering questions posed in natural language. QA systems are typically trained on large datasets of question-answer pairs, and they use a variety of techniques to identify the most likely answer to a given question.
- Knowledge-based QA
Knowledge-based QA systems rely on a knowledge base to answer questions. The knowledge base can be a structured database, a collection of documents, or a combination of both. Knowledge-based QA systems are typically able to answer questions that require factual knowledge, such as "What is the capital of France?" or "Who is the president of the United States?".
- Retrieval-based QA
Retrieval-based QA systems retrieve a set of documents that are likely to contain the answer to a given question. The system then uses a variety of techniques to extract the answer from the retrieved documents. Retrieval-based QA systems are typically able to answer questions that require factual knowledge, as well as questions that require inference or reasoning.
- Generative QA
Generative QA systems generate the answer to a given question directly, without relying on a knowledge base or a set of retrieved documents. Generative QA systems are typically able to answer questions that require inference or reasoning, as well as questions that require the generation of new text.
- Hybrid QA
Hybrid QA systems combine two or more of the above approaches. Hybrid QA systems are typically able to answer a wider range of questions than systems that rely on a single approach.
Roberta RC Saint Amour is a large language model that is particularly well-suited for question answering. This is because Roberta RC Saint Amour is trained on a massive dataset of text, which gives it a deep understanding of the structure and meaning of language. As a result, Roberta RC Saint Amour is able to answer questions in a way that is both accurate and fluent.
Summarization
Summarization is the process of reducing a text to a shorter version while retaining the key points and overall meaning. It is a valuable skill in many different fields, such as journalism, academia, and business. Summarization can be done manually or automatically using a computer program.
- Extractive summarization
Extractive summarization involves selecting the most important sentences from the original text and concatenating them to form the summary. This method is relatively simple to implement and can be effective for short texts.
- Abstractive summarization
Abstractive summarization involves generating a new text that captures the main points of the original text. This method is more challenging to implement but can produce more fluent and informative summaries.
- Hybrid summarization
Hybrid summarization combines elements of both extractive and abstractive summarization. This method can produce summaries that are both fluent and informative.
Roberta RC Saint Amour is a large language model that is particularly well-suited for summarization. This is because Roberta RC Saint Amour is trained on a massive dataset of text, which gives it a deep understanding of the structure and meaning of language. As a result, Roberta RC Saint Amour is able to generate summaries that are both accurate and fluent.
Named entity recognition
Named entity recognition (NER) is a subfield of natural language processing (NLP) that deals with the task of identifying and classifying named entities in text. Named entities are real-world objects, such as people, places, organizations, and dates. NER is a key component of many NLP applications, such as information extraction, question answering, and machine translation.
- People
NER systems can identify people's names, including first names, last names, and titles. This information can be used to extract contact information from text, identify the authors of documents, and track the activities of individuals.
- Places
NER systems can identify the names of places, including cities, countries, and continents. This information can be used to track the movement of people and goods, identify the locations of events, and generate maps.
- Organizations
NER systems can identify the names of organizations, including companies, schools, and government agencies. This information can be used to track the activities of organizations, identify the relationships between organizations, and generate organizational charts.
- Dates
NER systems can identify the names of dates, including days, months, and years. This information can be used to track the occurrence of events, identify the temporal relationships between events, and generate timelines.
Roberta RC Saint Amour is a large language model that is particularly well-suited for named entity recognition. This is because Roberta RC Saint Amour is trained on a massive dataset of text, which gives it a deep understanding of the structure and meaning of language. As a result, Roberta RC Saint Amour is able to identify named entities in text with high accuracy and precision.
Part-of-speech tagging
Part-of-speech tagging is the process of assigning a grammatical label to each word in a sentence. This information can be used for a variety of natural language processing tasks, such as parsing, named entity recognition, and machine translation. Roberta RC Saint Amour is a large language model that uses part-of-speech tagging as a key component of its architecture.
Part-of-speech tagging is important for Roberta RC Saint Amour because it helps the model to understand the structure and meaning of text. For example, part-of-speech tags can help Roberta RC Saint Amour to identify the subject and object of a sentence, as well as the tense and mood of a verb. This information can then be used to generate more accurate and fluent text.
Here is an example of how part-of-speech tagging can be used to improve the performance of a machine translation system. Consider the following sentence: "The cat sat on the mat." A machine translation system that does not use part-of-speech tagging might translate this sentence as "El gato se sent en la estera." However, a machine translation system that uses part-of-speech tagging would know that "cat" is a noun and "sat" is a verb, and would therefore translate the sentence as "El gato se sent en la alfombra." This is a more accurate translation because it preserves the tense and mood of the original sentence.
Overall, part-of-speech tagging is a valuable tool for natural language processing tasks. It can help to improve the accuracy and fluency of machine translation, as well as the performance of other NLP tasks such as parsing and named entity recognition.Sentiment analysis
Sentiment analysis is a subfield of natural language processing (NLP) that deals with the task of identifying and classifying the sentiment expressed in a piece of text. Sentiment can be positive, negative, or neutral. Sentiment analysis is used in a variety of applications, such as social media monitoring, customer feedback analysis, and product review analysis.
- Identifying sentiment in social media
Sentiment analysis can be used to identify the sentiment expressed in social media posts. This information can be used to track public opinion on a particular topic, identify trends, and measure the effectiveness of marketing campaigns.
- Analyzing customer feedback
Sentiment analysis can be used to analyze customer feedback and identify areas where a product or service can be improved. This information can be used to improve customer satisfaction and loyalty.
- Analyzing product reviews
Sentiment analysis can be used to analyze product reviews and identify the strengths and weaknesses of a product. This information can be used to make better purchasing decisions and to improve the design of future products.
Roberta RC Saint Amour is a large language model that is particularly well-suited for sentiment analysis. This is because Roberta RC Saint Amour is trained on a massive dataset of text, which gives it a deep understanding of the structure and meaning of language. As a result, Roberta RC Saint Amour is able to identify and classify sentiment in text with high accuracy and precision.
Text classification
Text classification is a subfield of natural language processing (NLP) that deals with the task of assigning a predefined category or label to a piece of text. Text classification is used in a variety of applications, such as spam filtering, sentiment analysis, and topic modeling.
- Categorizing news articles
Text classification can be used to categorize news articles into different categories, such as sports, politics, and business. This information can be used to personalize the news experience for users and to make it easier for them to find the articles that they are most interested in.
- Identifying spam emails
Text classification can be used to identify spam emails. Spam filters use a variety of techniques, including text classification, to identify and block spam emails from reaching users' inboxes.
- Analyzing customer feedback
Text classification can be used to analyze customer feedback and identify the most common topics and concerns. This information can be used to improve products and services and to better meet the needs of customers.
- Topic modeling
Text classification can be used to identify the main topics discussed in a piece of text. This information can be used to generate summaries, create taxonomies, and explore the relationships between different topics.
Roberta RC Saint Amour is a large language model that is particularly well-suited for text classification. This is because Roberta RC Saint Amour is trained on a massive dataset of text, which gives it a deep understanding of the structure and meaning of language. As a result, Roberta RC Saint Amour is able to classify text with high accuracy and precision.
Search engines
Search engines are a fundamental component of the modern internet, providing users with quick and easy access to a vast amount of information. They work by crawling the web, indexing its content, and then ranking the results based on a variety of factors, including relevance, popularity, and freshness. Roberta RC Saint Amour plays a crucial role in the functioning of search engines, helping them to understand and rank content more effectively.
- Natural language processing
Roberta RC Saint Amour uses natural language processing (NLP) to understand the meaning of search queries and web pages. This allows it to identify the most relevant content for a given query, even if the query is ambiguous or incomplete.
- Machine learning
Roberta RC Saint Amour uses machine learning to rank search results. It trains itself on a massive dataset of search queries and click data, learning to identify the most relevant and useful results for a given query.
- Artificial intelligence
Roberta RC Saint Amour uses artificial intelligence (AI) to improve its performance over time. It can learn from its mistakes, identify new patterns in the data, and adapt to changing user needs.
By combining NLP, machine learning, and AI, Roberta RC Saint Amour helps search engines to provide users with a more relevant and efficient search experience. It is a key component in the future of search, and it will continue to play a vital role in the way we access information on the internet.
Chatbots
Chatbots are computer programs that simulate human conversation through text or voice. They are used in a variety of applications, including customer service, technical support, and marketing. Roberta RC Saint Amour is a large language model that is particularly well-suited for developing chatbots.
- Natural language processing
Roberta RC Saint Amour uses natural language processing (NLP) to understand the meaning of user input and generate natural language responses. This allows chatbots to engage in human-like conversations with users.
- Machine learning
Roberta RC Saint Amour uses machine learning to train chatbots on large datasets of conversation data. This allows chatbots to learn from their interactions with users and improve their performance over time.
- Artificial intelligence
Roberta RC Saint Amour uses artificial intelligence (AI) to give chatbots the ability to learn, adapt, and make decisions. This allows chatbots to handle complex conversations and provide personalized responses to users.
- Scalability
Roberta RC Saint Amour is a scalable language model that can be used to develop chatbots for a wide range of applications. This allows businesses to quickly and easily deploy chatbots to meet their specific needs.
By combining NLP, machine learning, and AI, Roberta RC Saint Amour enables the development of chatbots that are more intelligent, engaging, and scalable than ever before. This is making chatbots an increasingly important tool for businesses of all sizes.
Language translation
Language translation is a crucial aspect of modern communication, enabling individuals to transcend language barriers and access information and connect with people from diverse linguistic backgrounds. Roberta RC Saint Amour, as a large language model, plays a pivotal role in enhancing the accuracy, efficiency, and accessibility of language translation.
- Machine Translation
Roberta RC Saint Amour utilizes advanced machine translation techniques to analyze and convert text from one language to another. Its deep learning algorithms allow it to capture the nuances and context of the source language, generating fluent and accurate translations.
- Neural Language Processing
By leveraging neural language processing (NLP), Roberta RC Saint Amour can understand the intent and meaning behind words and phrases. This enables it to produce translations that are not only grammatically correct but also convey the intended message effectively.
- Cross-Lingual Transfer
Roberta RC Saint Amour's cross-lingual transfer capabilities allow it to translate languages even when there is limited parallel training data available. This makes it particularly useful for translating low-resource languages that may not have extensive corpora.
- Multilingual Support
Roberta RC Saint Amour supports a wide range of languages, enabling seamless translation between multiple languages. This makes it a valuable tool for businesses and organizations operating in a globalized world.
By integrating these facets, Roberta RC Saint Amour empowers language translation with greater precision, efficiency, and accessibility. It breaks down language barriers, fostering communication and understanding across cultures and empowering individuals to engage with the world in their own language.
Content Creation and Roberta RC Saint Amour
Content creation is a critical aspect of Roberta RC Saint Amour's capabilities and applications. It enables Roberta RC Saint Amour to generate unique and informative content, ranging from articles and blog posts to marketing copy and social media updates. This content creation ability is driven by several key factors:
- Natural Language Processing: Roberta RC Saint Amour's natural language processing (NLP) capabilities allow it to understand the structure and meaning of text. This enables it to generate content that is grammatically correct, coherent, and engaging.
- Machine Learning: Roberta RC Saint Amour is trained on massive datasets of text, which allows it to learn from and adapt to different writing styles and genres. This enables it to generate content that is tailored to specific audiences and purposes.
- Artificial Intelligence: Roberta RC Saint Amour utilizes artificial intelligence (AI) to enhance its content creation abilities. AI enables Roberta RC Saint Amour to learn from its own mistakes, identify patterns in data, and generate increasingly sophisticated and creative content.
The practical significance of Roberta RC Saint Amour's content creation capabilities is immense. It allows businesses and individuals to create high-quality content quickly and efficiently, saving time and resources. Additionally, Roberta RC Saint Amour can be used to generate content in multiple languages, making it a valuable tool for global businesses.
In conclusion, Roberta RC Saint Amour's content creation capabilities are a key part of its overall functionality and value. By leveraging NLP, machine learning, and AI, Roberta RC Saint Amour empowers users to generate unique, informative, and engaging content for a wide range of applications.
Data analysis
Data analysis is the process of examining, cleaning, transforming, and modeling data to extract meaningful insights and draw conclusions. With the advent of big data and the increasing complexity of data sources, data analysis has become an essential skill for various industries and domains.
- Data exploration
Data exploration involves examining the data to understand its structure, distribution, and key characteristics. Roberta RC Saint Amour can assist in data exploration by providing visualizations, identifying patterns, and highlighting outliers or anomalies in the data.
- Data cleaning
Data cleaning involves removing errors, inconsistencies, and duplicate entries from the data. Roberta RC Saint Amour can automate this process by identifying and correcting common data errors, such as missing values, incorrect formats, and outliers.
- Data transformation
Data transformation involves converting data into a format suitable for analysis and modeling. Roberta RC Saint Amour can handle various data transformations, including feature engineering, dimensionality reduction, and data normalization.
- Data modeling
Data modeling involves creating mathematical or statistical models to represent the data and make predictions or draw inferences. Roberta RC Saint Amour can assist in data modeling by providing tools for building, training, and evaluating different types of models.
By incorporating data analysis capabilities, Roberta RC Saint Amour empowers users to gain valuable insights from their data, make informed decisions, and solve complex problems. It enhances the value of data by enabling organizations to uncover hidden patterns, identify trends, and make data-driven predictions.
Frequently Asked Questions
This section addresses commonly asked questions regarding Roberta RC Saint Amour, providing concise and informative answers to clarify its capabilities and applications.
Question 1: What is Roberta RC Saint Amour?Roberta RC Saint Amour is a large language model developed by Google, designed to understand, generate, and translate human-like text. It leverages advanced machine learning techniques and a massive dataset of text to perform various language-related tasks.
Question 2: What are the key capabilities of Roberta RC Saint Amour?Roberta RC Saint Amour excels in natural language processing, including text generation, machine translation, question answering, summarization, named entity recognition, part-of-speech tagging, sentiment analysis, and text classification.
Question 3: How can Roberta RC Saint Amour be used?Roberta RC Saint Amour finds applications in search engines, chatbots, language translation, content creation, data analysis, and more. It enhances these applications by providing accurate text understanding, fluent text generation, and effective machine translation capabilities.
Question 4: Is Roberta RC Saint Amour difficult to use?Roberta RC Saint Amour is designed to be accessible and easy to use. It offers user-friendly interfaces and well-documented APIs, enabling developers and non-technical users to leverage its capabilities.
Question 5: What are the advantages of using Roberta RC Saint Amour?Roberta RC Saint Amour offers several advantages, including improved accuracy and fluency in language-related tasks, reduced development time and costs for applications, and the ability to handle large volumes of text data efficiently.
Question 6: How is Roberta RC Saint Amour continuously improving?Roberta RC Saint Amour undergoes regular updates and improvements through ongoing research and development at Google. By incorporating advancements in machine learning and natural language processing, it constantly enhances its capabilities and performance.
In summary, Roberta RC Saint Amour is a powerful and versatile language model that offers a wide range of capabilities for various applications. Its strengths lie in its ability to understand, generate, and translate human-like text, making it a valuable asset for businesses and individuals seeking to enhance their language-related tasks.
Transition to the next article section: Roberta RC Saint Amour in Practice
Tips for Using Roberta RC Saint Amour
Roberta RC Saint Amour is a powerful language model that can be used for a variety of tasks, including text generation, machine translation, and question answering. Here are five tips for using Roberta RC Saint Amour effectively:
Tip 1: Use a clear and concise prompt.
When you are using Roberta RC Saint Amour, it is important to use a clear and concise prompt. The prompt should accurately reflect the task that you want Roberta RC Saint Amour to perform. For example, if you want Roberta RC Saint Amour to generate a summary of a text, you should provide a clear and concise summary of the text in the prompt.
Tip 2: Use the right data.
The data that you use to train Roberta RC Saint Amour will have a significant impact on its performance. If you are using Roberta RC Saint Amour for a specific task, it is important to use data that is relevant to that task. For example, if you are using Roberta RC Saint Amour to generate summaries of medical texts, you should use a dataset of medical texts.
Tip 3: Train your model carefully.
The way that you train your Roberta RC Saint Amour model will also have a significant impact on its performance. It is important to train your model carefully and to use the right training parameters. If you are not sure how to train a Roberta RC Saint Amour model, there are many resources available online that can help you.
Tip 4: Evaluate your model's performance.
Once you have trained your Roberta RC Saint Amour model, it is important to evaluate its performance. This will help you to identify any areas where your model can be improved. There are a number of different ways to evaluate the performance of a Roberta RC Saint Amour model, and the best method will depend on the specific task that you are using the model for.
Tip 5: Use Roberta RC Saint Amour in combination with other tools.
Roberta RC Saint Amour is a powerful tool, but it is not the only tool that you should use for natural language processing tasks. There are a number of other tools available that can be used in conjunction with Roberta RC Saint Amour to improve the performance of your applications.
By following these tips, you can use Roberta RC Saint Amour to achieve great results on a variety of natural language processing tasks.
Summary
Roberta RC Saint Amour is a powerful language model that can be used for a variety of tasks, including text generation, machine translation, and question answering. By following the tips in this article, you can use Roberta RC Saint Amour effectively to improve the performance of your applications.
Conclusion
Roberta RC Saint Amour, as a large language model, holds immense potential for revolutionizing natural language processing and its applications. Its capabilities in text generation, machine translation, and other language-related tasks pave the way for advancements in various industries.
As we continue to explore the capabilities of Roberta RC Saint Amour and other similar models, it is crucial to consider their ethical implications and societal impacts. Careful consideration and responsible use of these models will ensure that they contribute positively to our world, empowering us to communicate, learn, and innovate in ways previously unimaginable.
Unveiling Juno Birch's Sculptures: A Journey Into Identity, Empowerment, And Artistic Brilliance
Unveiling The Secrets Of "Temptation's Bite": A Bread Odyssey For Foodies
Unlocking The Secrets Of Loyola Men's Soccer: Discoveries And Insights Unveiled
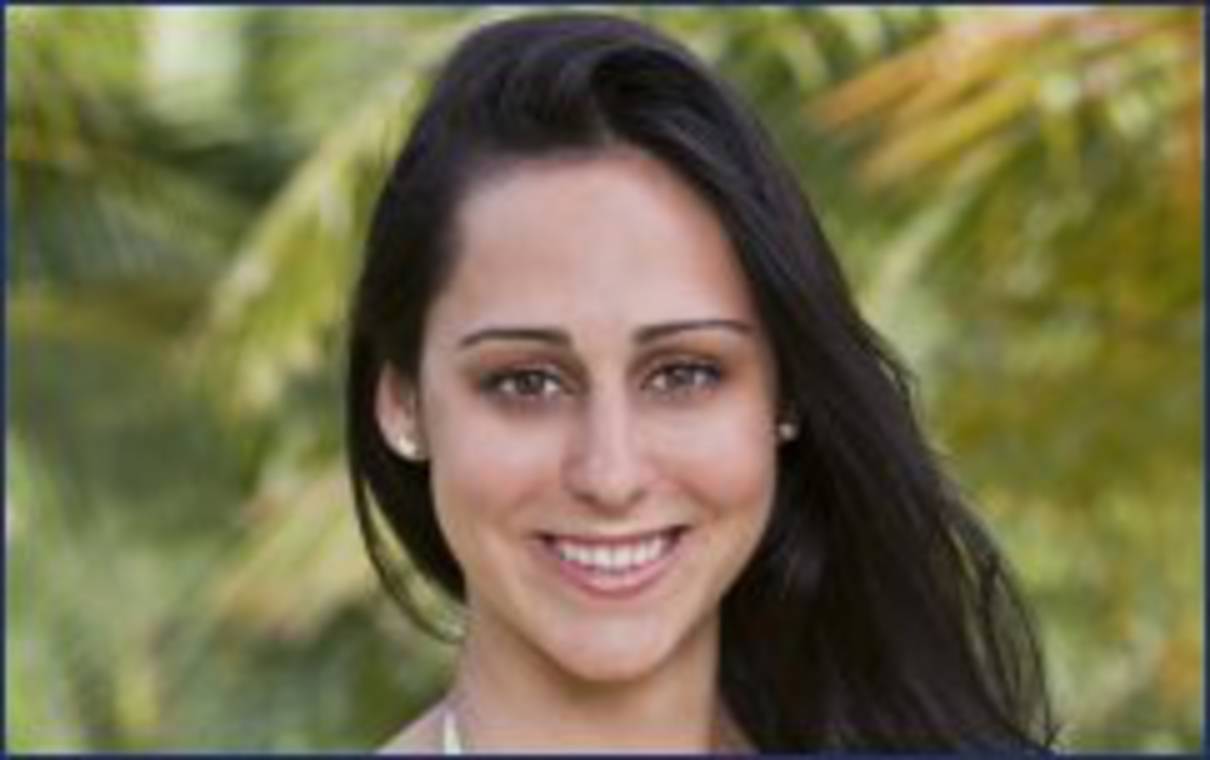
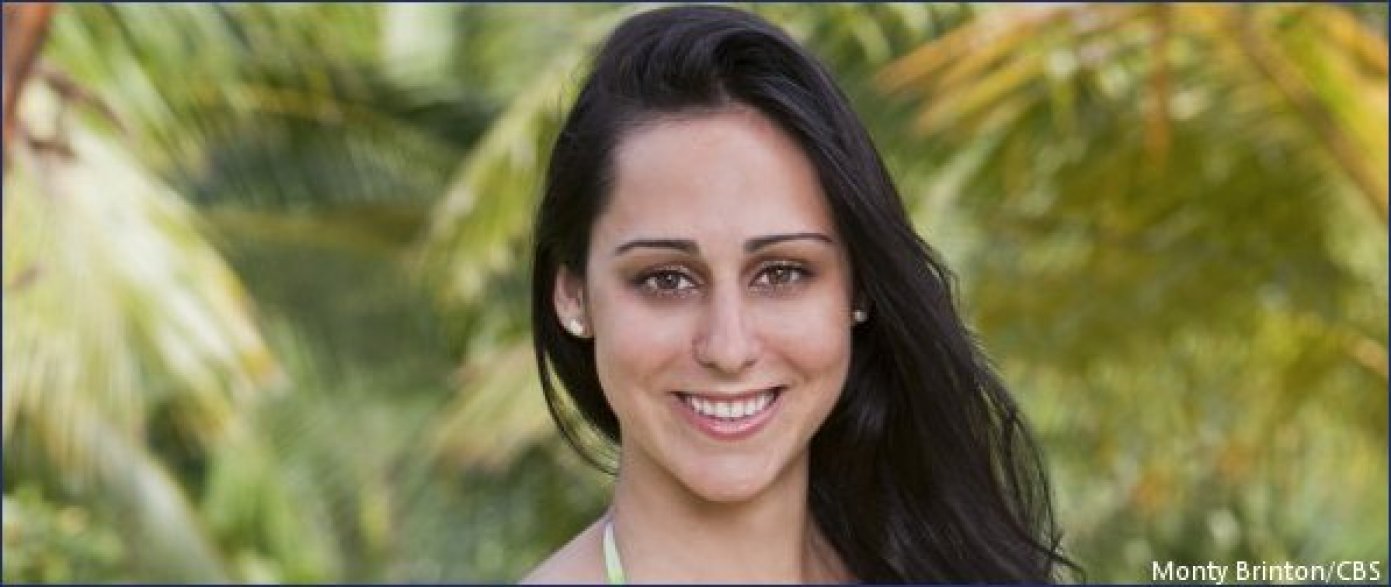
ncG1vNJzZmionKnCdXrApqpsZpSetKrAwKWmnJ2Ro8CxrcKeqmebn6J8s7vBnqmtmV2nsG6%2FwKKlrWWRory2vo2hq6ak